RESEARCH
Purpose of the Process & Data Intelligence (PDI) research unit is to study and develop technologies that support the formalization, retrieval, and usage of knowledge pertaining (business) processes and the healthcare and wellbeing world. Current research efforts pertain:
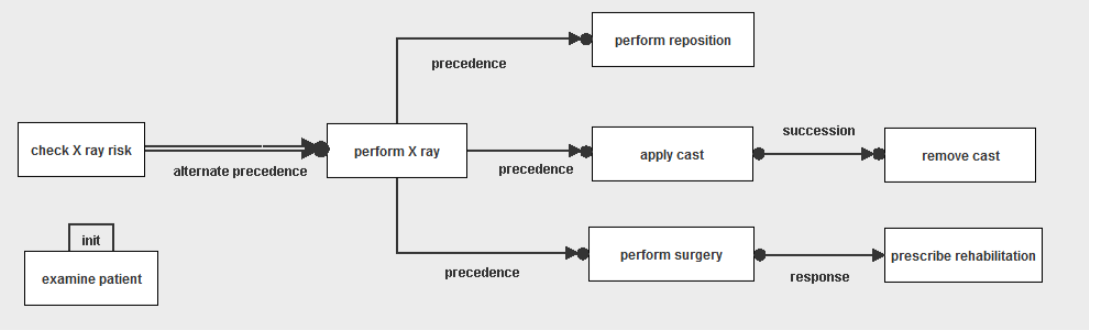
Process Discovery & Deviance Mining
The aim of process discovery is to build a process model from an event log without prior information about the process. We are working towards the discovery of different types of BUsiness Porcess models starting from different types of data sources. Examples are: The discovery of Hybrid Process Models composed of a mixture of informal causal graphs and formal Petri Nets; the discovery of (Declarative) Process Models built from positive and negative execution traces; the discovery of (Procedural) Process Models from text; and the discovery of (Declaratve) discriminative patterns between positive and negative execution traces.
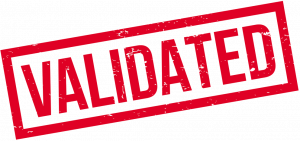
Process Verification and Conformance Checking
We are intersted in providing theoretically sound, yet practically viable support for the verification of data aware procedural process models. In particular we have exploited planning techniques to perform reachability analysis, and conformance checking also in the event of partial execution traces. The latter problem has been explored also with the usage of abduction. We are also interested in exploiting formal verificatin techniques, such as the computation of unsat cores, to support the improvement of Declare models.
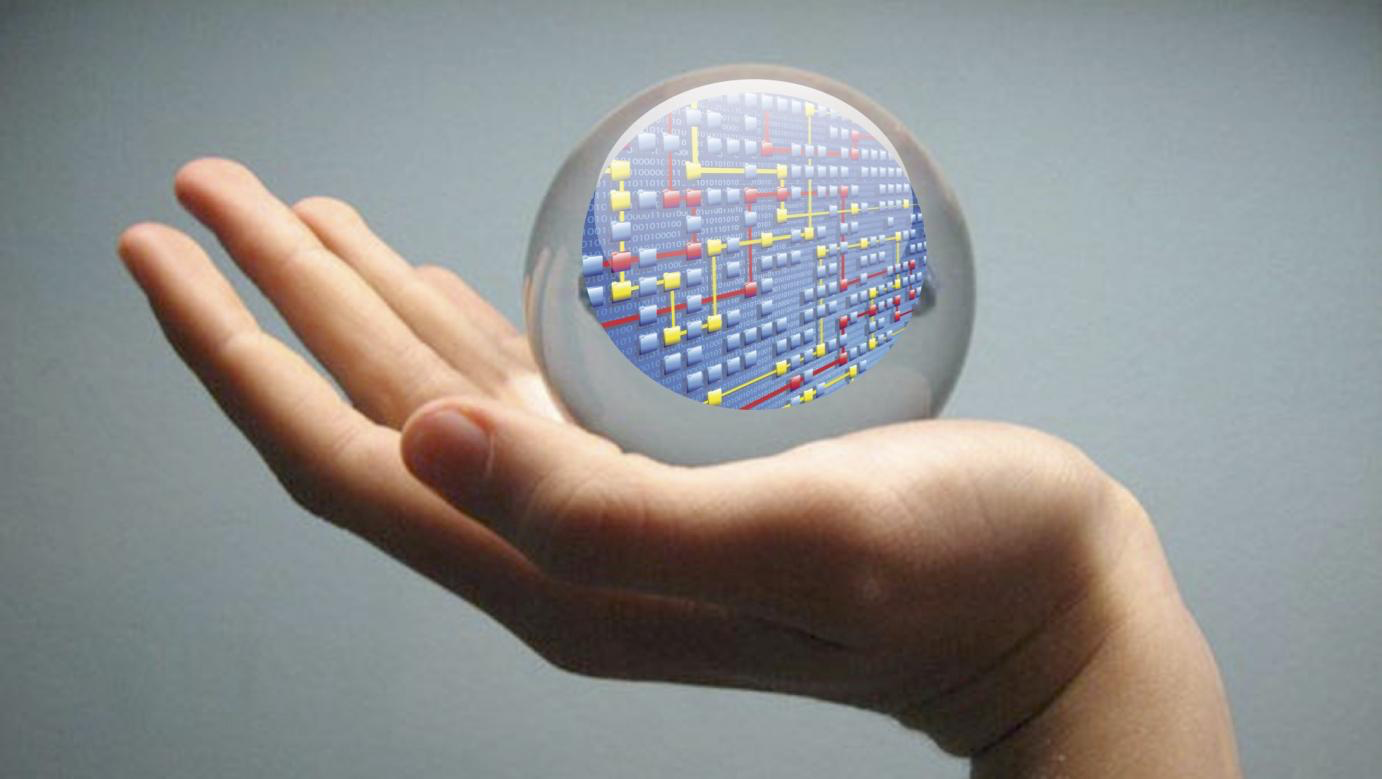
Predictive Process Monitoring
Modern information systems that support complex business processes generally maintain significant amounts of process execution data, particularly records of events corresponding to the execution of activities (event logs). In this reserach line we investigate techniques and support the Nirdizati tool that exploit event logs to predict how ongoing (uncompleted) cases will unfold up to their completion. We work on a wide series of prediction tasks using different types of Machine Learnig techniques, including the insertion of background knowledge. Recent work aims at supporting explainable prediction both in terms of improving the techniques and assess their usefulness with end users.
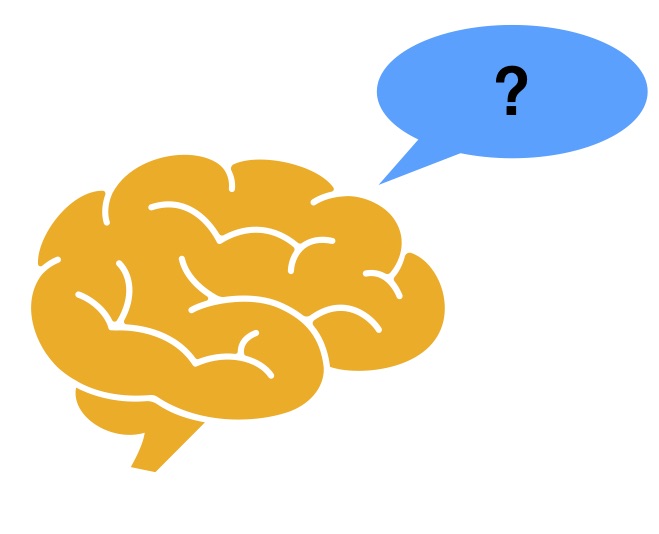
Explainable AI FOR PREDICTIVE PROCESS MONITORING
The complexity of modern AI techniques and the sensitivity of the decision-making task, nonetheless, requires these techniques to be robust and trustworthy. We are interested in investigating explainable AI techniques (XAI) to support transparent predictive process monitoring, especially by adapting and evaluating counterfactual explanation generation techniques to the specifics requirements of Process Monitoring tasks.
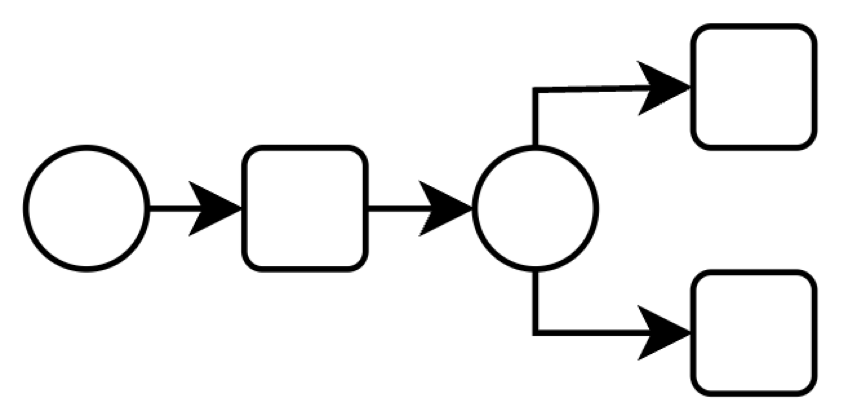
Business Process Simulation
Business process simulation (BPS) provides a widely used and flexible approach to analyse and improve business processes. It exploits a process simulation model, that is, a process model extended with additional information for a probabilistic characterization of the different run-time aspects such as case arrival rate, task durations, routing probabilities, resource utilization, and so on, to produce a significantly large number of process runs. Statistics over these runs are then collected to gain insight into the process, and to determine the possible issues such as bottlenecks, wastes, or costs. Through simulation experiments, also, various ‘what if’ questions can be answered, and different process redesign alternatives can be compared with respect to key performance indicators of interest. We are interested in studying BPS as a way to optimize the process and also support artificial intelligence techniques, particularly the Reinforcement Learning model.
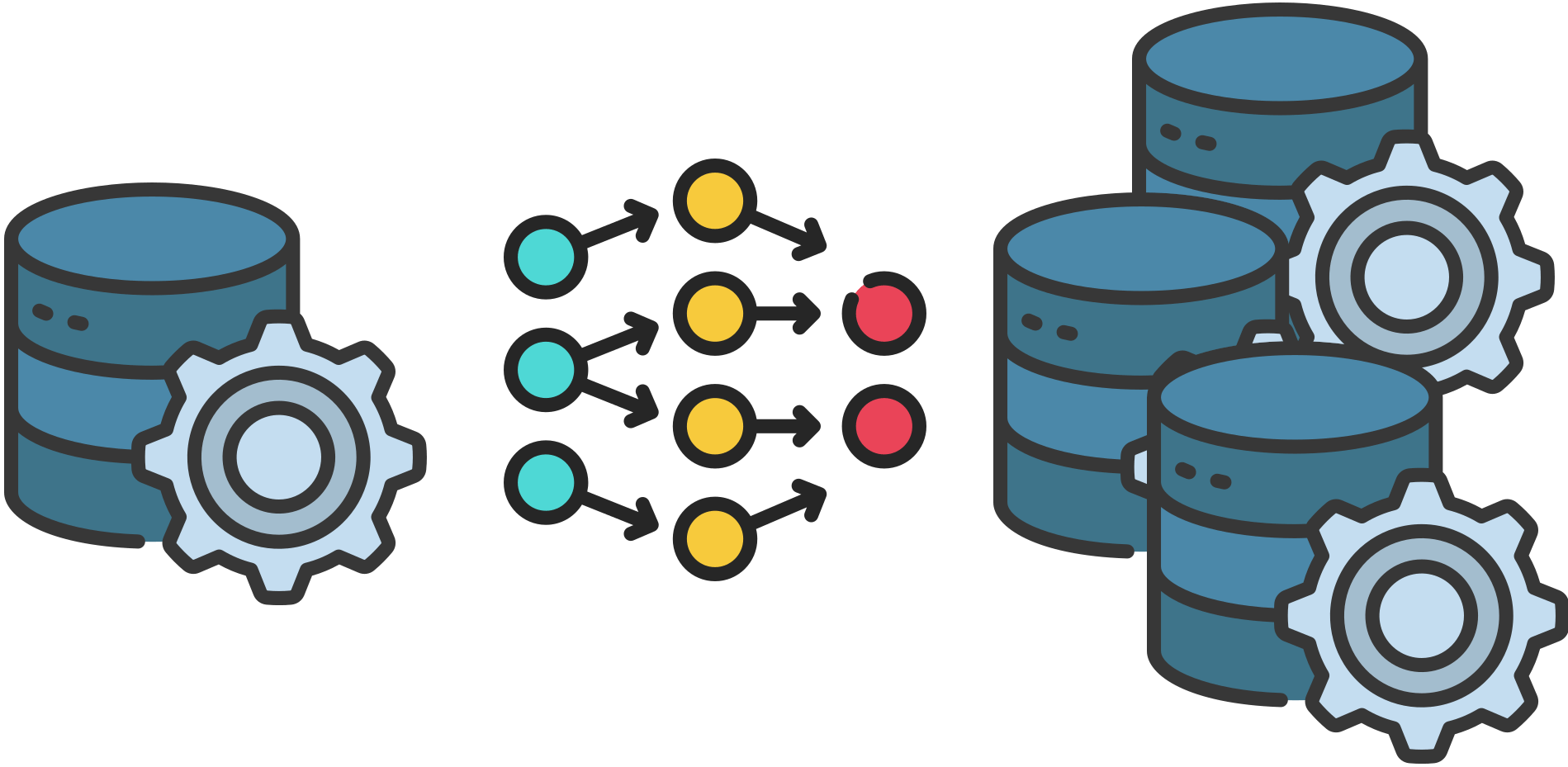
Generative models for business processes
In recent years, generative AI models have achieved remarkable success across various fields, including process mining. At our research unit, we focus on applying generative neural networks—particularly conditional variational autoencoders (CVAE)—to accurately capture and reproduce the complexities of business process data. These generative models enable innovative applications in process mining, such as data augmentation, which enriches existing datasets; what-if scenario analysis, allowing businesses to explore potential process changes; and data anonymization, ensuring privacy while preserving the utility of process data.
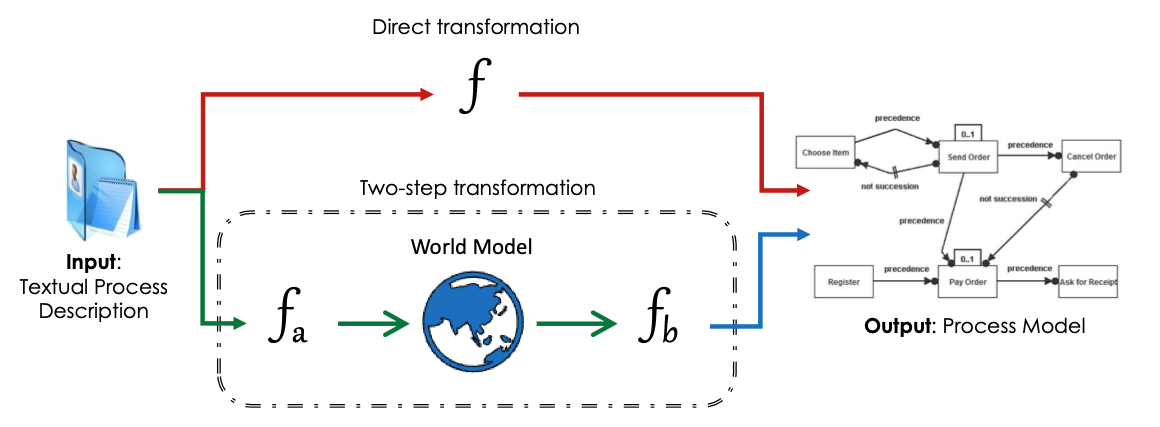
PROCESS MODEL EXTRACTION FROM TEXT
The initial elicitation of a process model from documents is a time-consuming and cost-intensive operation. Typically, these documents provide unstructured descriptions of how a process is performed in a company, outlining the necessary activities to achieve the desired outcome rather than the specific activities executed in a particular process instance from start to finish. This distinction makes it impractical to apply process mining techniques in this context. This extraction task is complex since it requires analyzing natural language descriptions while simultaneously taking care of the multiple linguistics levels (syntactical, semantics, and pragmatics) and mitigating ambiguities. To overcome these issues, we propose a novel approach to address these issues by leveraging the power of Large Language Models (LLMs) and generative AI to support the automatic construction of knowledge graphs representing process models, starting from the LLM predictions.